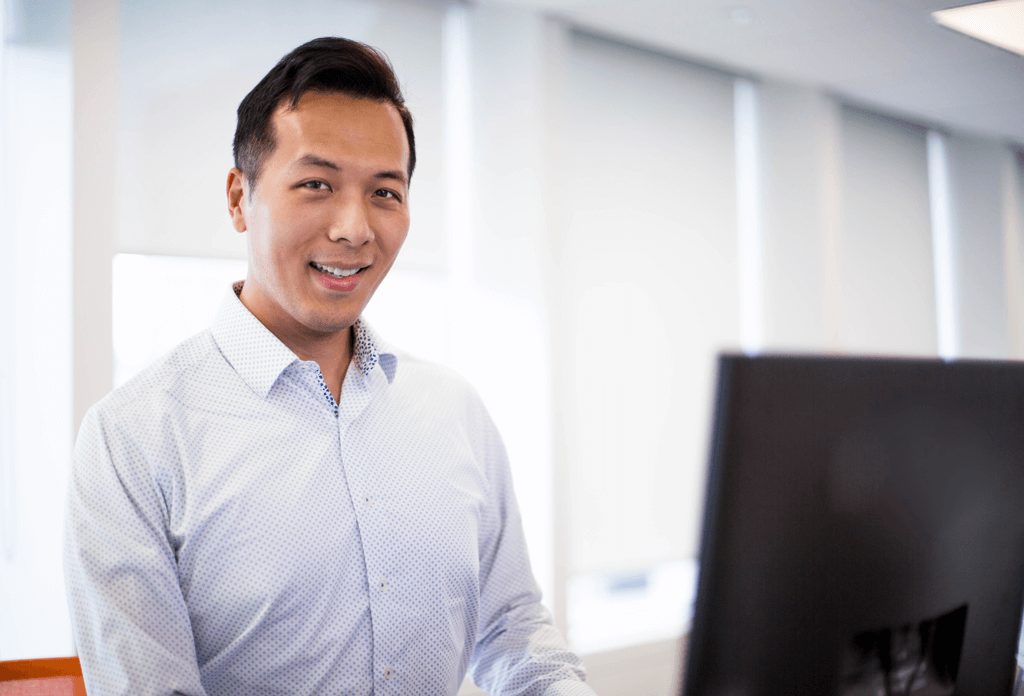
Six Lessons Learned Bringing a Machine Learning Product to Market
Editor’s note: The article below was written by David Vuong, Product Lead, Business Intelligence, Predictive Analytics and User Experience at Cority and originally appeared on the Georgian Growth Network. David shares his experience in bringing an analytics product to market.
Cority builds Environmental, Health, Safety and Quality (EHSQ) software solutions to advance our customers’ journeys to sustainability and operational excellence. While our products have been highly successful, we’ve always known that the value of the data we collect would be a game-changer, for several reasons:
- We made the move to a SaaS model ahead of the competition, so we’ve had more time to collect more data and it’s readily available for us to do our research;
- We serve enterprise clients, so our data is global in scale; and
- We focus on protecting workers and the environment, so delving into the data presented a compelling use case: helping clients manage risk and prevent problems before they occur.
The impact of collaboration
When Georgian invested in Cority, one of the first meetings we had was an Impact Workshop with Madalin Mihailescu, Georgian’s CTO, and his team. The goal of the meeting was to identify high-value/low-cost opportunities to leverage our data and create additional value for our clients. To that end, we focused on:
- Identifying the intersection between Cority’s capabilities and the market’s problems,
- Ways to improve workplace safety, since these solutions can have the most direct impact on life and limb and
- Instances where the data we have is amenable to analysis.
Working together, we developed a project plan that included hiring staff to build the models and identifying clients to validate potential scenarios. As a result of our collaboration, we now offer Cority Analytics—a product that enables clients to predict employees’ probability of injury, ensure safety programs exceed peer benchmarks and rapidly model “what if” scenarios.
As anticipated, Cority Analytics has been a hit. It was named Operational Health and Safety’s 2018 Product of the Year and recently received a 2019 Industrial Safety and Hygiene News (ISHN) Readers’ Choice award, which recognizes the industry’s best occupational health and safety products.
Lessons learned along the way
We’ve certainly learned a lot from the inception of the product to where we are today. Here are six key takeaways that may help other product managers or teams who are bringing an analytics product to market.
1. Anticipate data challenges
With analytics projects, there’s data everywhere but not everything may be as it seems or in the right format. For example, because our EHSQ software solutions are configurable, we struggled with standardized data. To solve the issues we were facing, we worked with the Georgian Impact Team on a side initiative that included looking at external standards for reporting injuries.
2. Build your team to meet your objectives
We initially underestimated the time it would take for our data scientists to understand the challenges we faced—and didn’t think we needed a data engineer on the team. My advice in hindsight? Hire a data engineer in tandem with a data scientist so these two roles can learn in parallel. While the scientist focuses on the nature of issues and problems, the engineer can be working on the architecture, and data security and privacy. This approach can streamline the process significantly.
3. Put the right product manager (PM) in place
For an analytics project, the PM needs to wear multiple hats. To identify challenges and implement changes, the PM must understand the language of data science and how data science research happens. But they should also speak the language of the business and be able to articulate the big picture to the data scientists—translating the company’s and the users’ objectives to inform their research.
4. Think about go-to-market from the get-go
It’s possible to do so many exciting things with the data that it’s easy to get carried away. Make sure you’re solving real problems, not just interesting ones. Early adopters or an advisory board can be extremely helpful when you’re validating whether user challenges require new solutions.
Remember that packaging and positioning also matter. You might want “your baby” to be its own product, but it might fit better as an add-on to an existing product. Thinking about workflow and how customers will use the product will guide the right decisions.
5. Expand your job description
Cutting-edge analytics projects don’t necessarily follow the defined timelines of traditional software development projects. As product manager, be prepared to spend more time explaining your approach to the organization and setting expectations accordingly.
You’ll also need to flex your entrepreneurial muscles by taking risks, being scrappy and fighting for the resources you need. And you’ll need to get good at sales and develop your own go-to-market materials. The earlier you can outline the value proposition for your new product, the greater impact you’ll have on the product’s success.
6. Shake your pom-poms
A new analytics product can cause a cultural shift across the business. To get everyone on the same page, you’ll need to be the product cheerleader for both internal and external audiences.
It also helps to have senior leadership drumming up support alongside you. At Cority, we were lucky to have the support of the Georgian team, who really believed in the product, but it could be any executive sponsor who shares your vision.
To read more about how Cority built the market-leading safety analytics product, read our case study.
Read more like this
Generative AI: Opportunities and Challenges for Startups
As we discussed in our previous post, the sudden rise of generative AI…
Cloud Spend Management: A Guide for Startups
Over the past several months, CoLab executives and customers have told us…
Cybersecurity Lessons Learned Using Machine Learning for Anomaly Detection
At Georgian, we invest in high-growth technology companies that harness the power…