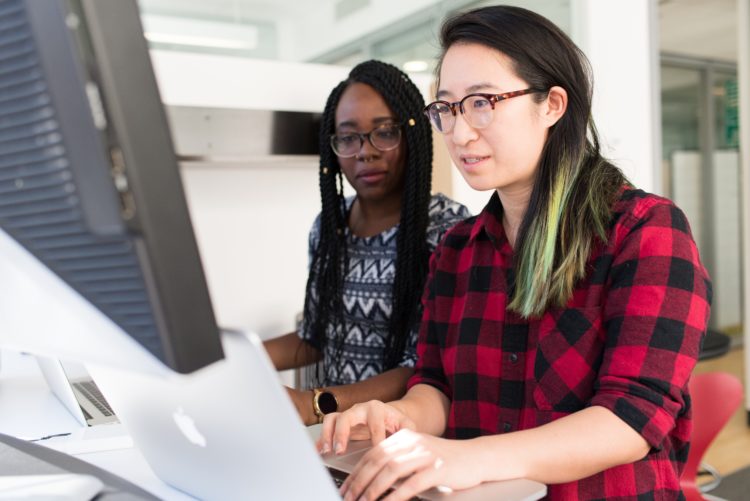
Getting Buy-in for Your AI Product
July’s installment of the Scaletech AI Product Series featured Mike Olson, founder and former CEO of Cloudera and Nahla Salem, Senior Product Manager at Loopio alongside co-hosts Alistair Croll, founder of Fwd50, Startupfest and Scaletech, and Dr. Theresa Johnson, Data Scientist at Airbnb and angel investor. The focus for this event was, “How do I choose the right opportunities for AI, and how do I get organizational buy-in for an AI product?” Below, we share five insights from the event to help you drive organizational alignment around your first AI product.
1. Create a Narrative: Why Does This Matter to the Customer?
To get buy-in for any product, the first step is to build a narrative that shows why it matters to your customers and the lift it will deliver to your business. As a Data Scientist or AI Product Manager, you then have to sell this narrative to get the team aligned around your vision. Your colleagues might be excited about the new tech and what it can do, but your role is to keep the discussion focused on the user and the benefits.
Should you do anything differently for an AI product at this stage? Not necessarily. If you take a user-centric approach, the difference between creating a narrative around an AI product and a non-AI product might not be that drastic. When you’re adding AI, all you really want to do is accentuate or speed up how your users realize the product’s value.
You’ll be able to create a stronger narrative if you first take time to understand and map out all the processes your users accomplish when using your product. Once you’ve done this, prioritize the most valuable opportunities to automate or augment a step in the process.
2. Don’t Go Looking for Nails
It’s important not to fall in love with AI for AI’s sake. When you have expertise on the team, it’s tempting to look for ways to use that expertise. As the saying goes, when you have a hammer, everything looks like a nail.
When you’re focused on the customer, though, the technology you’re using should become less important. As Nahla Salem says: “At the end of the day, your users have deadlines. [They]…have zero interest in your technology, but they are very interested in how you are going to help them do their jobs more quickly.”
In other words, your users don’t care about the latest machine learning techniques and algorithms. They care about how you’re going to solve their problems.
From a user’s perspective, it shouldn’t matter, nor should it be obvious whether you’re even using AI or not. Even if you’re using advanced machine learning techniques, the UI should still be as simple as possible. As DJ Patil says, “no new feature should add training time to the user.”
3. Embrace Open Source
The buy vs. build debate is still relevant for AI products. Do your diligence on whether it makes sense to buy and white-label an off-the-shelf solution. If you do go down the build route, open-source libraries provide a gold mine of resources.
Many folks in the machine learning (ML) community come from academia, where there is a long history of collaborating on research and sharing results to help the field as a whole.
This ethos means that there’s a thriving open-source community around machine learning. Open source can provide a boost to teams that are just getting started and more experienced ML teams alike. For example, by sharing your models, others may improve them for new use cases that you would not have seen otherwise. Georgian takes this approach with our own ML toolkits, like Foreshadow and TensorFlow Privacy.
Smart people, wherever they are, whatever sector they’re working in, can come together to collaborate on a shared infrastructure that they care about. More and more of that infrastructure is shared. More and more of the differentiation is at a higher level: service delivery, UI, and so on… My default were I starting a business today, would be to rely on open source.
– Mike Olson, co-founder and founding CEO of Cloudera
4. ML Augments Human Decision Making
One of the barriers you commonly hear about getting buy-in for ML-driven products is that people believe that automation will replace their job, so they obstruct machine learning projects. Your customers may feel this way too, so it’s essential to consider this properly.
As the world moves towards data-led services, the simple fact is that you need machine learning to make sense of the sheer volume of data. Humans simply can’t process the volumes of data we deal with today. Your job when selling your AI project is to be clear that ML doesn’t remove human decision making from the equation, it improves our ability to make decisions.
This is especially true when making complex business decisions that rely on more factors that humans can consider at the same time. Companies that use ML need visionary managers and smart people to help make those ML-informed decisions.
Well-designed ML feeds decision making by people.
Mike Olson
5. Build with your User in Mind
If you’re in the business of helping your users to make ML-informed decisions, you’ll need to give them the right data to support their typical thought process.
This begins with recognizing that human decisions are not always on/off. Neither are the decisions reached by algorithms. The best AI products communicate uncertainty and provide extra information to help their users deal with uncertainty. The context you deliver alongside your insights, and the confidence thresholds you are able to expose play a crucial role. Often, you’ll need to take the time to understand these parameters before you can get buy-in. You can read about some best practices for providing these insights here.
If you’re making a decision for the consumer, how do you teach them what factors went into that decision?
Dr. Theresa Johnson
Take, for example, vehicle maintenance. You’re designing a system that monitors a vehicle’s systems and alerts drivers when systems are likely to fail. If the system detects a potential fault with your brakes, you would want to let drivers know about it, even if the uncertainty was high. Whereas if it was a fault with, say, the AC, you might want to set a higher certainty threshold before alerting the driver.
In the end, how you present your insights, along with error and uncertainty, will depend on your users and the use case. When done right, these supporting features can be truly valuable to your users.
Sign Up for Scaletech
The Scaletech AI Product Series is an invite-only event series designed for scale-stage entrepreneurs who are building AI and Machine Learning (ML) into their products. In August, we’ll be covering AI product design and tooling–everything from building a top-notch ML pipeline to managing mass amounts of data.
If you enjoyed this read and you’d like to be invited to the rest of the series, shoot katie@georgian.io an email.
Read more like this
Generative AI: Opportunities and Challenges for Startups
As we discussed in our previous post, the sudden rise of generative AI…
Cloud Spend Management: A Guide for Startups
Over the past several months, CoLab executives and customers have told us…
Cybersecurity Lessons Learned Using Machine Learning for Anomaly Detection
At Georgian, we invest in high-growth technology companies that harness the power…